This blog post was authored by Hannah Tarver and Steven Gentry, members of the Digital Library Assessment Interest Group’s Metadata Assessment Working Group (DLF AIG MWG). It is intended to provide a summary overview of metadata assessment in digital libraries, including its importance and benefits.
If you are interested in metadata evaluation, or want to learn more about the group’s work, please consider attending one of our meetings!
Metadata assessment involves evaluating metadata to enhance its usefulness for both internal and external users. There are three main categories of metadata:
[1] Administrative metadata provides information about the management or preservation of digital objects such as when it was archived, what access or restrictions are placed on an item, a unique/permanent identifier for an object, when files were last migrated/copied/checked, etc.
[2] Descriptive metadata is the human-readable text describing the creation and content of an item, such as who made it, what it is about, and when it was made/published. This information is displayed in a publicly-accessible and searchable user interface (while administrative and structural metadata may be less visible, or only internally accessible).
[3] Structural metadata names all of the files associated with an item (e.g., a single PDF or multiple individual image files, metadata files, OCR files, etc.) and describes the relationship among them. For example, if there are images for individual text pages, or multiple views of a physical object, the structural metadata would express how many images there are and the order in which they should be displayed.
Specific pieces of information may be stored in different types of metadata depending on a local system (e.g., some access information may be in public-facing descriptive metadata, or some preservation information may be incorporated into structural metadata). An organization could evaluate various characteristics for any or all of these metadata types to ensure that a digital library is functioning properly; however, most researchers and practitioners focus on descriptive metadata because this information determines whether users can find the materials that fit their personal or scholarly interests.
Metadata Errors
Metadata assessment is necessary because errors and/or inconsistencies inevitably creep into records. Collections are generally built over time, which means that many different people are involved in the lifecycle of metadata; standards or guidelines may change; and information may be moved or combined. There are a number of different quality aspects that organizations may want to evaluate within metadata values; here are some examples:
Accuracy
- Typos. Spelling or formatting errors may happen by accident or due to a misunderstanding about formatting rules. Even when using controlled lists, values may be copied or selected incorrectly.
- Mis-identification. Metadata creators may incorrectly name people or places represented or described in an item. This is especially problematic for images.
- Wrong records. Depending on how items and their metadata records are matched in a particular system, a record describing one item may be applied to an entirely different item (see Figure 1).
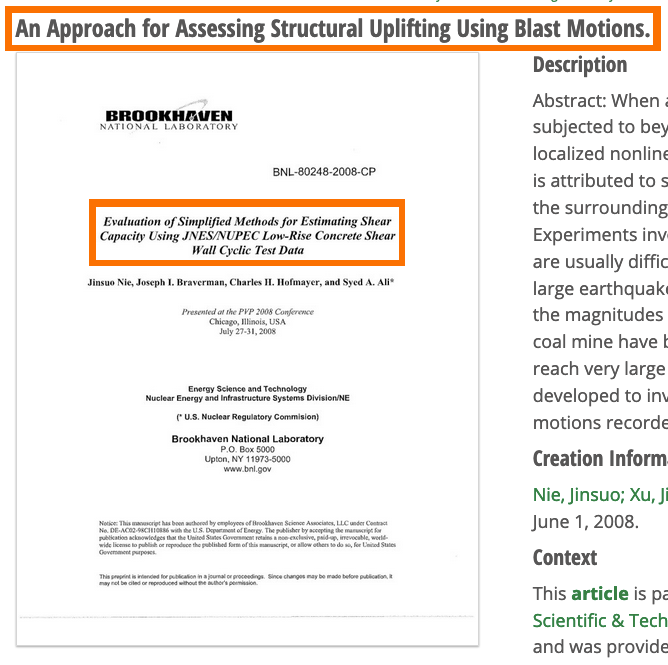
Completeness
- Missing information. Whether due to a lack of resources or simply by accident, information may be left out of metadata records. This could include required data that affects system functionality, or optional information that could help users find an item.
- Unknown information. Especially for cultural heritage objects—such as historic photos and documents—information that would benefit researchers (e.g., detailing the creation of an item or important people/locations) may be absent (see Figure 2).
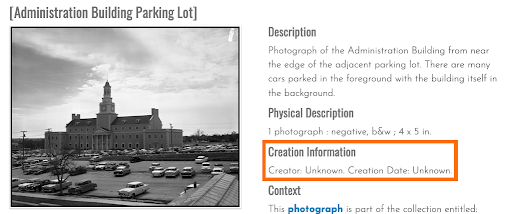
Conformance to expectations
- Inappropriate terminology. Sometimes, the language used in records does not align with the terms that a primary user-group might prefer (e.g., a subject value for “kittens” instead of “felines” in a science database record). This may be due to an inconsistent use of words (e.g., “cars” vs. “automobiles”) or an editor’s lack of knowledge about the most appropriate or precise descriptors (e.g., “flower brooch” for corsage, or “caret-shaped roof” for gabled roofs).
- Outdated language. Collections that describe certain groups of people—such as historically underrepresented or marginalized groups—may use language that is inappropriate and harmful. This is particularly relevant for records that rely on slow-changing, commonly shared vocabularies such as Library of Congress Subject Headings (see Further Reading, below).
Consistency
- Formatting differences. If exact-string matching is important, or if fields use controlled vocabularies, any differences in formatting (e.g., “FBI” vs. “F.B.I.”) might affect searching or public interface search filters.
- Name variations. The same name may be entered differently in different records depending on how they are written on items (e.g., “Aunt Betty” vs. “Beatrice”), name changes (e.g., maiden names or organizational mergers), information available over time, or inconsistent use of a name authority (see Figure 3).
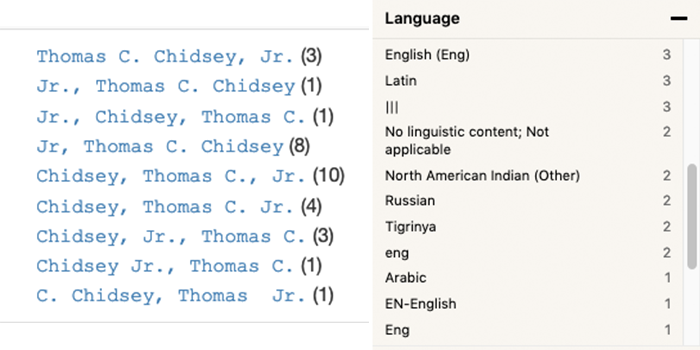
Timeliness
- Legacy or Harvested Data. If formatting rules have changed over time, or if information has been migrated or imported from another system, inconsistent values or artifacts may be present in the records. These include MARC subdivisions in name/subject values (see Figure 4), technical mark-up from databases (e.g., “. pi. /sup +/, p”), or broken character encodings (e.g., “& # 39;” [spaces added for this example] instead of an apostrophe).

Benefits
There are a number of benefits to users and organizations when metadata is assessed and improved over time. For example:
Users:
- Records with complete, accurate, and consistent metadata are more findable in online searches.
- Similarly-described materials allow relevant items to be more easily collocated.
- Good metadata may allow public interfaces to enhance the user experience (e.g., via filtering search results).
Organizations maintaining digital collections:
- Error-free metadata is easier to migrate from one system to another or to integrate with other resources (e.g., a discovery layer).
- Complete records make it easier for staff to find and promote/advertise special items when opportunities arise.
- Well-formed metadata records are more easily shared with other organizations (e.g., the Digital Public Library of America), thereby making such materials more widely accessible.
- Good records reflect well on the organization, as users might be put off by spelling, grammar, or related issues.
Methods/Resources
Although metadata assessment is tremendously beneficial, it often requires organizational support such as a large or ongoing commitment of people and other resources. First and foremost, knowledgeable personnel are crucial to successful assessment and metadata improvement; trained professionals contribute metadata expertise (e.g., ability to determine what values need to be reviewed or changed) and subject-area specialties necessary for successful metadata assessment efforts (particularly for larger projects). Additionally, assessment and mitigation or enhancement of collections require significant personnel time to evaluate and edit metadata.
Another major component of metadata assessment activities are tools, which may include spreadsheet-based resources (e.g., OpenRefine), or specialized scripts written in a variety of programming languages. An important note to bear in mind is that even though tools can expedite metadata assessment efforts, they may require technical experience and training to be used effectively.
Aside from using tools for broad analysis, one popular assessment method is manually evaluating records (i.e., looking at an individual record and reviewing all of the values). Employing this kind of workflow would appeal to professionals for a few reasons:
- Manual metadata assessment requires the least amount of technological training.
- Particularly for smaller collections, checking all values in a record may allow for fewer edits & revisions (i.e., records are not “touched” as often).
- Some aspects of metadata quality (e.g., accuracy) can only be determined through manual evaluation.
However, there are challenges to consider when assessing metadata. Effective manual evaluation, for example, can be hard to scale as records increase, and may not provide collection-level insights. Additionally, as collections grow in size, comprehensive assessment becomes more difficult and requires increased resources to review and correct errors or outdated values. Finally, it is important to recognize that improving records is an ongoing and often iterative process. Overall, metadata assessment is a resource-balancing exercise.
Further Reading
This blog post was informed by numerous resources and practical experience. The following resources provide additional information if you want to learn more about various aspects of metadata assessment:
Papers/Publications
- Noted by members of DLF AIG MWG as an early influential work regarding quality:
Bruce, Thomas R., and Diane I. Hillmann. (2004). The continuum of metadata quality: Defining, expressing, exploiting. https://ecommons.cornell.edu/handle/1813/7895. - Overview comparing metadata assessment frameworks and metrics:
Ochoa, X., & Duval, E. (2009). Automatic evaluation of metadata quality in digital repositories. International Journal on Digital Libraries, 10(67). https://doi.org/10.1007/s00799-009-0054-4 - White paper documenting the results of the MWG’s benchmarks survey about how organizations evaluate their own metadata:
Gentry, S., Hale, M. L., Payant, A., Tarver, H., White, R., & Wittmann, R. (2020). Survey of Benchmarks in Metadata Quality: Initial Findings. http://dlfmetadataassessment.github.io/assets/WhitePaper_SurveyofBenchmarksinMetadataQuality.pdf - An overview about equitable, anti-oppressive, and inclusive metadata: Sunshine State Digital Network. (2020). Introduction to conscious editing series. Retrieved February 23, 2021, from https://sunshinestatedigitalnetwork.wordpress.com/2020/09/16/introduction-to-conscious-editing-series/
- A bibliography of resources about harmful description as it pertains to archival description: Digital Collections and Archives. (n.d.) Additional reading: Potentially harmful language in archival description. Retrieved February 23, 2021, from https://dca.tufts.edu/about/policies/Additional-Reading-Potentially-Harmful-Language-in-Archival-Description
Metadata Assessment Working Group Resources
- Environmental scan documenting publications, presentations, tools, and organizations related to assessment: http://dlfmetadataassessment.github.io/EnvironmentalScan
- Metadata Assessment Framework & Guidance: http://dlfmetadataassessment.github.io/Framework
- Metadata Assessment Workshop materials, which intended to introduce “basic skills and knowledge needed to assess metadata quality using data analysis tools”: http://dlfmetadataassessment.github.io/MetadataWorkshop
Example images come from the Digital Collections at the University of North Texas (UNT) — https://digital2.library.unt.edu/search — and from the Digital Public Library of America (DPLA) — https://dp.la/