This blog post was authored by members of the Digital Library Assessment Interest Group’s Metadata Assessment Working Group (DLF AIG MWG),
If you are interested in metadata evaluation, or want to learn more about the group’s work, please consider attending one of our meetings!
Introduction
The DLF Assessment Interest Group Metadata Working Group (MWG) collaborates on projects related to a number of metadata quality and assessment issues (https://wiki.diglib.org/Assessment:Metadata). The metadata quality aspects discussed in this post were formulated by Bruce & Hillmann (2004) and also used as the basis of the Metadata Assessment Framework formulated by the MWG: https://dlfmetadataassessment.github.io/framework
Organizations assess metadata in their digital collections in a number of different ways. This post is intended to provide a case study to show how three universities evaluate metadata in their digital library systems as an example for other institutions that may be interested in similar assessment activities.
Organizations
Colgate University
System. Colgate currently uses Islandora 7, and is closely watching institutions like Whitman College who are actively migrating to Islandora 8. We migrated our main digital collections from CONTENTdm about 4 years ago and are still migrating our institutional repository from Bepress’ Digital Commons.
Size. Colgate’s digital collections (https://digitalcollections.colgate.edu/) contain nearly 6000 individual objects, but a count of book and newspaper pages increases the number to 112,781. An additional 500 items in our institutional repository will come online soon.
Editors. Students create or capture basic descriptive and administrative metadata for the majority of our Special Collections/University Archives materials. One librarian then adds subject-related metadata, and checks the descriptive metadata for accuracy and conformance to our local standards before upload. Three paraprofessional catalogers have been working on two historical collections as remote work during the pandemic, which is also reviewed by the same librarian before upload. Work on assessing and creating metadata and migrating the Colgate institutional repository has been shared by two librarians and one paraprofessional cataloger.
Assessment. Most of our larger assessment projects have been tied to migration: either as we migrated out of CONTENTdm or Digital Commons. Now that we are seeing how some of the metadata is working together (or not working, as the case may be), we are doing targeted assessment and resultant amelioration of our prestige and most used collections.
University of North Texas
System. At the University of North Texas (UNT) Libraries, our digital library system was built in-house and is maintained by our team of programmers, so we can make changes and additions when needed.
Size. The Digital Collections (https://digital2.library.unt.edu/search/) currently house more than 3.1 million items/metadata records, hosted locally within our archival system. All materials are described using the same schema (UNTL), which has 21 possible fields including 8 that are required for every item.
Editors. Most metadata creation is handled by students who are employed and trained in UNT Libraries departments, or Libraries staff. Also, many of our materials come from partner institutions that own the physical collections, so staff or volunteers at those locations often have access to edit records in their collections. On a weekly basis, we usually have around 50 people editing records in our system.
Assessment. At UNT, we have ongoing assessment at a number of levels, including research, which has been published in a number of places. Additionally, in 2017 we implemented three integrated tools (count, facet, cluster) that allow any editor to review metadata at a collection level or systemwide (a paper from when we implemented them: https://digital.library.unt.edu/ark:/67531/metadc1248352/). These tools reorganize metadata values and information about the records to assist in identifying records that require correction (or just review), but an editor still has to manually edit each record. As a project is completed, an editor will generally use the tools to do general quality control of their own work; they are also used to identify various system-wide problems that can be addressed as time permits.
- COUNT allows an editor to see the number of entries for a field or field/qualifier combination (e.g., how many records have 0 subjects, or 3 subjects, or 1,000 subjects; how many records have 1 series title or 15 series titles; etc.).
- FACET displays the unique values for a field or field/qualifier combination, which is particularly useful for finding typos or seeing which values are most common in a collection (e.g., most popular keywords).
- CLUSTER has two functions that work across all values in a field or field/qualifier combination: [1] normalizes values based on a chosen algorithm (there’s a list of options), then displays any instances (clusters) where there are multiple unique strings that have the same normalized key (e.g., “John Smith” and “Smith, John” would both normalize to “john smith” in the default algorithm, so those would cluster together); or [2] sorts every value according to criteria (e.g., length or alphanumeric pattern) and groups together the values that have the same chosen quality (e.g., all the subject values that are 1 character long, or 3 characters long, or 500 characters long).
These tools have allowed us to identify and correct a large number of issues, however, we are still enhancing various parts of our system.
Whitman College
At Whitman, we are currently in the process of migrating from Islandora 7 to Islandora 8 as part of a LYRASIS IMLS grant which focuses on moving from Fedora 3 to Fedora 6. As a smaller institution, we have roughly 20,000 items and formed a Metadata Working Group to assess all things metadata. Members consist of our Scholarly Communications Librarian and Institutional Repository Manager, our Associate Archivist, and our Digital Assets and Metadata Librarian. The three of us have weekly group meetings and primarily use Google Sheets and Google Documents. In the beginning, the Metadata Working Group used Trello as well to help keep track of our metadata assessment and clean up, however as we’ve progressed and formalized our fields, we’ve moved away from it.
How do you assess accuracy?
Colgate. We have generally measured accuracy in two ways: are the record fields present (or blank) and are the values correct or appropriate for the material, i.e., this is or is not a photograph of Colgate President Ebenezer Dodge. The first we can assess in a mostly automated manner by using filters on our spreadsheets or Open Refine. The second can only be assessed by a human who has some familiarity with Colgate’s collections and history, which naturally takes much longer and is still subject to human error.
UNT. This generally requires manual assessment (e.g., to determine if the record values match the item), but depending on the problem, the count or facet tools may show where something doesn’t align with what we know about a collection. Also, facet is helpful to find mis-matches between values and qualifiers (e.g., a creator/contributor name labeled “organization” but formatted as a personal name or vice versa).
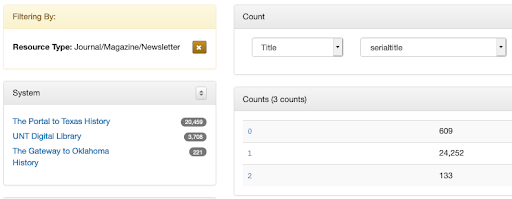
Whitman. Our repository is broken into several Main Collections, (Archives, Honors Theses, Faculty and Student Scholarship, Maxey Museum, and Sheehan Gallery) . Each Main Collection has its own measure of accuracy, however, during the past year, our Metadata Working Group created guidelines to help unify our metadata and create a structural accuracy that would be of most help upon our migration. We began our assessment on each field, comparing the field and metadata use across all collections. In this process, we created standards for our fields, and evaluated the clean up that needed to happen in order for our legacy metadata to follow the new standards. Once decisions were made, it was up to the three of us to manually make the changes, and check our metadata for accuracy.
How do you assess completeness?
Colgate. We have different, but informal standards of completeness depending on whether the item resides in our digital collections or in our institutional repository. For our digital collections, which are generally used by students or faculty looking for items on a topic or theme, we have 15 standard fields that we use, and all are required if appropriate metadata exists or can be determined. In our digital repository, which contains university sponsored research, patrons are generally looking for a ‘known item’, and their searches are more straightforward on titles, course titles/numbers, or authors. In our IR, we use 10 fields, and consider the record complete if we have or can capture or develop metadata for each of them. After we migrate to Islandora 8, we also hope to add a drupal form onto the front of our IR where we can have submitters deposit their own research and accompanying metadata. Submissions would not be accepted or deemed ‘complete’ unless all of the required fields are completed with the appropriate metadata chosen from a drop down list.
UNT. We have a formal definition of “minimally-viable records” (https://library.unt.edu/digital-projects-unit/metadata/minimally-viable-records/) which includes a metric to calculate completeness based on values in 8 fields required for every record: (main) title, language, (content) description, 2 subjects, resource type, format, collection, and institution (i.e., the partner department or organization that owns the items). Our system automatically calculates “completeness” and displays it in the item record — both a summary view and with color-coded notations in the editing form so that editors can see which fields are missing — and on the main Dashboard so that editors can limit their view to just complete/incomplete records.

Additionally, we can use the count tool to find fields that may be missing for particular material types or collections. For example, we don’t require “creator” values, but if we are looking at a collection of theses/dissertations or published books, a record that has a creator count of 0 would need to be reviewed. Similarly, materials from our Libraries’ Special Collections always include a “citation” that references the archival collection, even though this is rarely used for materials from other partners.
Whitman. It’s hard to pin-point when exactly our metadata assessment is complete when preparing for our migration as we’re constantly learning new things about Islandora 8, and adapting our metadata. However during our initial metadata assessment, we used a spreadsheet to track changes. It was a good place to indicate that a change (such as a field being removed) was completed and what changes still needed to be implemented. This came in hand specifically as we worked on several fields at once, and had up to 80 individual spreadsheets that needed to be worked on.
How do you assess conformance to expectations?
Colgate. Colgate switched from CONTENTdm to Islandora because of patron complaints about the system, and how difficult items were to discover. Whether that was because the metadata was non-standard or the interface was not user-friendly was not clearly documented. In a previous iteration of our institutional repository, items could be self-uploaded with any value in any field, or even incomplete information, so there was very little conformance to either internal or external expectations. A combination of Excel filters, individual assessment, or fresh creation of metadata was all that could ensure any conformity for our IR.
We have learned that users like to search by subject in our more ‘historical’ collections, so we try to include at least one subject field for each described item. In the case of our regular digital collections, we use Library of Congress Subject Headings, while our institutional repository uses a combination of other standards such as JEL codes for economics papers or author supplied keywords.
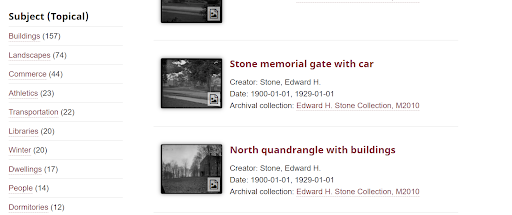
For internal expectations, we have a formal best practices document that all metadata creators must use that provides standardized formats for names, dates, locations, collections names, rights management, and so on. The occasional filtering or faceting of spreadsheets and MODS datastreams allows us to check general compliance with the best practices.
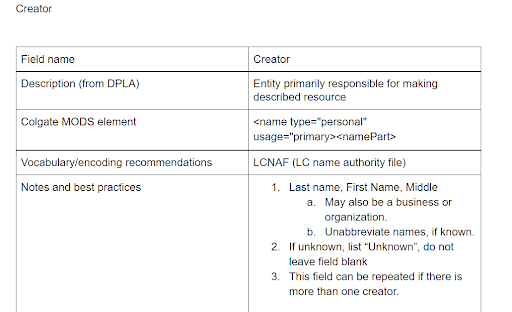
UNT. User/external expectations are difficult to evaluate and generally require manual proofreading, but we have started reviewing subject headings across various Libraries holdings/descriptions for ethical representation. Facet can be helpful for finding records that contain specific, problematic values.
In terms of internal expectations, structural metadata elements are built into our system, and we have extensive guidelines that document usage and formatting for all fields (https://library.unt.edu/digital-projects-unit/metadata/input-guidelines-descriptive/). Our evaluation tools help to identify values that do not meet formatting requirements, particularly related to authorities and controlled vocabularies. One of the new adjustments that has been added within the last year is validation for selected controlled subject vocabularies. A warning message displays in the edit form if someone enters one of these values incorrectly, but also highlights incorrect values in facet and cluster when limited to those qualifiers. This also applies to date values that do not align with EDTF formatting.
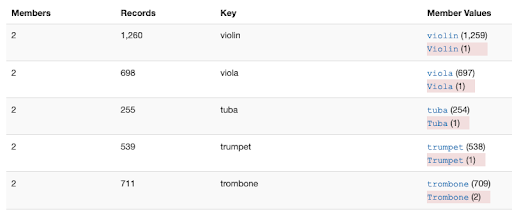
Whitman. When it comes to conformance to expectation it’s good to look at our Taxonomy Fields (field_linked_agent, field_subject, field_genre). As of now, our process is pretty labor intensive. First we take a field (field_subject for example) and copy the content from all our collections, pasting into a single spreadsheet tab. Then we would separate out using either Text to Columns (Excel) or Split into Columns (Google Sheets). This helped address repeated fields.
Once terms are separated, we manually add them to one column. From there we remove duplicates. Once duplicates are removed, we visually go down each cell to make sure our terms conform. This is a labor-intensive practice, however, it allows us to tie which collection the term came from, as well as create the opportunity for a drop-down list. For our relatively small item count and timeframe, it was easier to do in Google Sheets than OpenRefine.
How do you assess consistency?
Colgate. We are a joint metadata and cataloging department, so we use many of the same standards of uniformity with our non-MARC metadata as with our MARC metadata. The team of paraprofessional catalogers that create or edit non-MARC metadata finds it helpful to apply the rules of authority control and uniformity that we use in the ‘other half’ of our work. Combined with our Best practices for metadata core documents, we try to ensure that the way we format metadata is consistent across collections, and that we use our profiled fields consistently across multiple collections with very little variability.
We hope that our migration to Islandora 8 which allows for an ‘authority file’ style drop down list of terms will result in significantly less cleanup, particularly when it comes to names and geographical locations. We anticipate this will open up a broader range of metadata creation to our student workers, who will appreciate the convenience of picking a name or location from a list, which will make these terms accurate and in compliance, and less prone to typos or inconsistencies.
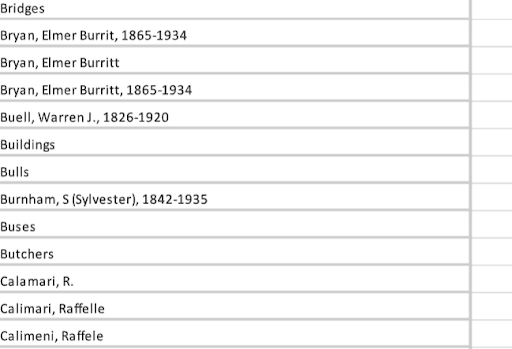
UNT. Generally we think of consistency as representing values in standardized ways to ensure that exact-string matching or searching for specific phrases could find all relevant items. Cluster and facet are both particularly useful to compare value formatting and usage for consistency. Within particular collections, consistency may also be evaluated in terms of whether fields are used in the same ways, which tends to show up in count.
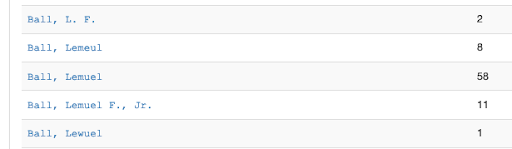
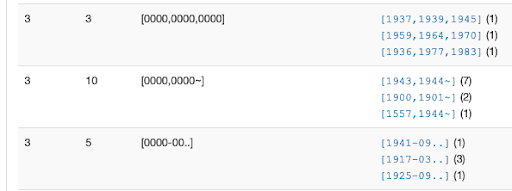
Whitman. The standards we created as a group included an in depth description as to what our metadata would look like going forward.
We also created a document that depicted examples of our metadata in each field and collection.
As we assessed our metadata remediating it so it conformed to our standards, we came across areas in which conforming was not possible (either it was not feasible, or the collection did not belong to the library). In those cases we indicated those exceptions in our documentation. Throughout our assessment, we continuously checked for consistency both between and within our collection spreadsheets manually. Much like checking for conformance of expectations, using spreadsheets and having multiple eyes checking for inconsistencies helped make sure they were consistent in both following our policies, but also consistent in the formatting of our metadata.
How do you assess provenance?
Colgate. We use Islandora, which supports storage of multiple versions of metadata. We can roll back metadata to previous versions in case of mistake, disaster, or re-assessment. It helps keep us honest when we can see who is responsible for the work being done, and also identify the legacy metadata (by creation date) that was performed by vendors, or migrated in from our CONTENTdm system.

UNT. We version all of our metadata and make the history/previous versions available to editors. Although we don’t generally assess this (except for a couple of papers comparing change across versions), it can be useful when doing other assessment or review activities to see who “touched” the record, what they changed, or when specific values/changes were introduced into a record. We also have the ability to “roll back” changes in extreme circumstances, although this isn’t something we have used.
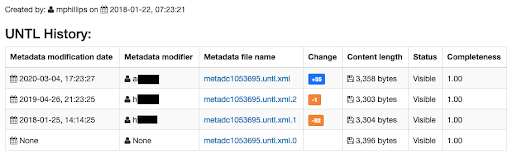
Whitman. We have future plans to assess and fine-tune the Provenance of our metadata. As of now, that information lives on archived spreadsheets across our shared Drive.
How do you define/measure timeliness?
Colgate. We used the migration of our institutional repository as a chance to peek at the timeliness and currency of our metadata and descriptions across collections. Some collections had been bouncing around for years, variously described in Dublin Core, MARC, or sometimes according to no schema at all. The IR is now fully MODS compliant, and it feels like we are on a strong foundation to accept and effectively describe new submissions in the fall. Looking more broadly, we are now sure that dates have been a particular problem in our history collections that were migrated from CONTENTdm. We plan to use Islandora’s filter option to look for missing date ranges for where we are confident we have coverage, and Open Refine to clean and standardize date-related metadata.
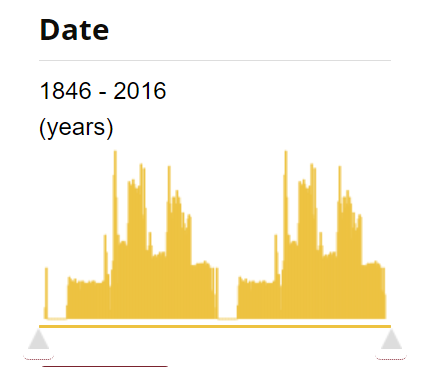
UNT. Our system contains a large amount of imported data from government databases and old values from early records that do not conform to current guidelines. Cluster and facet, in particular, help us to identify where values or formatting need to be updated. We can also use count to locate records that we might want to target for additional research (e.g., historical photos of the UNT campus that don’t have dates or locations that the wider community might be able to identify). Additionally, users often submit feedback about names, locations (including place points), or other information related to particular items that we can add to records.
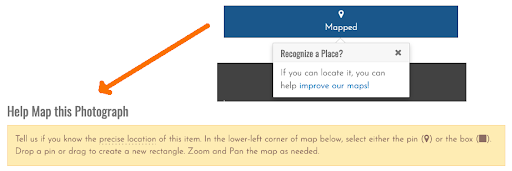
Whitman. When it comes to the timeliness of creating metadata, the process varies between the different Main Collections. For example, Faculty and Scholarship, and Archives tend to contain items that are likely to fall into the “timely ingest” category so classes can use their content. Theses, on the other hand, take a lengthy approach. First the theses are cataloged in OCLC, then the metadata is added to our repository. This process can take up to two months depending on our cataloger’s workload.
For our migration to Islandora 8, we started the remediation process in Summer of 2020 and are still fine-tuning it a year later. This lengthy process allowed us to assess our metadata and improve legacy descriptions. Though the clean up took a year, we acknowledge that it was time well spent as our new items will now have standards and examples to follow, making it easier and faster to add to our repository.
What challenges do you have evaluating metadata?
Colgate. Since we are a small department with a dual focus (metadata and cataloging) we are torn between balancing demands for creating new metadata for new collections, as well as improving and standardizing legacy metadata. Some of the most commonly requested changes (addressing missing or ill-formatted date metadata) are also the most difficult and time-consuming to fix, as they can not occur via a batch process. We are trying to target collection-by-collection assessment by prioritizing projects that improve the overall user experience, rather than just cleanup for cleanup’s sake.
Our general metadata review workflow is also not sustainable, with many creators and only one reviewer. On our wishlist is another metadata librarian who can focus on standards compliance at the point of collection creation, as well as training and supervising student workers.
UNT. Despite our evaluation tools, there are still issues that are difficult for us to find or change. For example, we can easily find unique values that exist across records, but not values that are duplicated in the same record. Also, editors still need to determine which values are correct and know the expectations for various items or collections, which requires quite a bit of manual review or correction. Additionally, some issues will always require an editor (or supervisor) to check metadata values against an item to verify accuracy or make a judgement call about how the item has been described within the bounds of our established guidelines and the context of the particular collection.
Another general concern is determining the best ways to prioritize and distribute resources to review and correct records across our system. Our edit tools help us to find potential issues system-wide, but, like all institutions, we have limited time and resources available to review or address those issues. Determining the most effective way to deploy editors or identify the “most important” issues is an area of ongoing research for us.
Whitman. We had a couple of challenges when it came to evaluating our metadata. First was the time in which we planned to evaluate and remediate our metadata. We were selected for the LYRASIS Grant in which our content would migrate from Islandora 7 to Islandora 8 around the time we were beginning our metadata assessment and remediation. This was challenging in the sense that there was a lot of information that needed to be remembered for not only the metadata remediation, but also to prepare for a MODS-RDF migration, as well as document the process in detail. Our Metadata Librarian often had multiple projects going on in a single day including planning the next fields to be assessed during our Metadata Working Group meetings while fixing fields that had just been assessed, while mapping all fields to RDF. Along with that there was some communication challenges where some group members referred to fields by their Public Lable, while others referred to them as their spreadsheet label (example: Access Statement” vs. access_condition).
The second challenge in evaluating metadata is spreadsheet versioning. We were lucky enough to have a sizable collection in which the work could be done in spreadsheets, however, it was difficult keeping track of various versions of spreadsheets and who/what work was done. Along with that, it was challenging to update each spreadsheet as we changed field names.